Ethical Considerations in AI: Addressing Bias and Ensuring Fairness
- Shriparna
- 12 June, 2023
- 2 mins ago
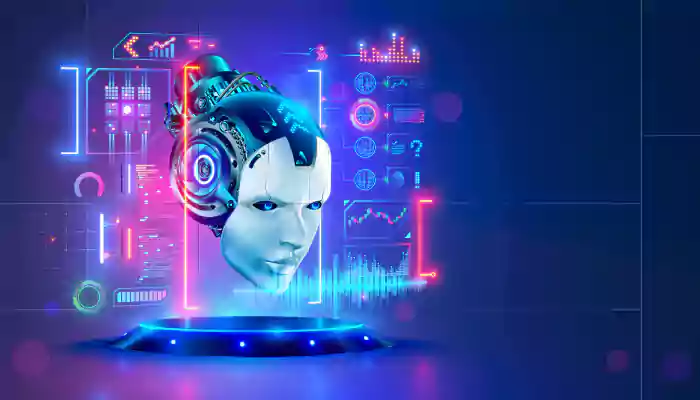
Ethical Considerations in AI: Addressing Bias and Ensuring Fairness
This article explores the importance of addressing bias in AI and the strategies required to ensure fairness.
Artificial Intelligence (AI) has revolutionized numerous industries, from healthcare to finance, by offering advanced algorithms and predictive capabilities. However, as AI becomes more prevalent in our daily lives, it is crucial to address the ethical considerations surrounding its development and deployment. One of the primary concerns is the presence of bias in AI systems, which can lead to unfair outcomes and perpetuate societal inequalities.
Understanding Bias in AI
Bias in AI refers to the systematic errors or prejudices that occur when developing or using AI algorithms. These biases can arise from various sources, including biased training data, flawed algorithms, or biased human decisions during the AI development process. When biased AI systems are deployed, they can perpetuate and even amplify existing societal biases, leading to discriminatory outcomes.Implications of Bias in AI
The implications of bias in AI can be far-reaching. For instance, biased AI algorithms used in recruitment processes may favour certain demographic groups, leading to discriminatory hiring practices. Similarly, biased AI in law enforcement can disproportionately target and impact marginalized communities.Strategies to Address Bias in AI
To mitigate bias and ensure fairness in AI systems, several strategies can be implemented:Diverse and Representative Data: Ensuring that the training data used to develop AI systems is diverse and representative of the population it aims to serve is crucial. This can be achieved by incorporating data from various sources and actively seeking input from underrepresented communities.
Transparent and Explainable Algorithms: It is important to develop AI algorithms that are transparent and explainable. This allows stakeholders to understand how decisions are made and identify potential biases. Increased transparency promotes accountability and helps build trust in AI systems.
Regular Auditing and Testing: Regularly auditing and testing AI systems for bias is essential. This involves evaluating the system's performance across different demographic groups and identifying any discrepancies or unfair outcomes. Such audits help in detecting and addressing biases promptly.
Ethical Guidelines and Regulations: Developing clear ethical guidelines and regulations for AI development and deployment is crucial. These guidelines should address issues such as data privacy, consent, and fairness. Regulatory bodies should ensure that AI systems comply with these guidelines and hold developers accountable for any biases or unfair practices.
It is essential for developers, policymakers, and stakeholders to collaborate in shaping the future of AI with a strong focus on ethics and social responsibility.